Rina Dechter, with Others, Receives a $5M NSF Grant to Improve AI-Based Causal Decision Making
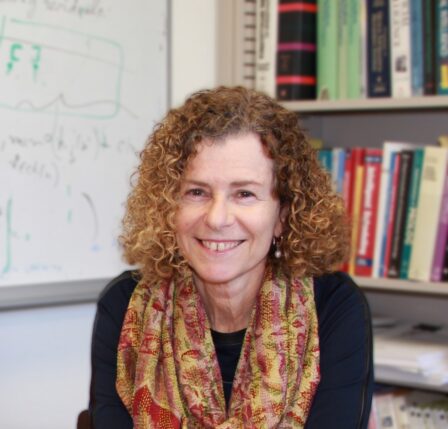
Computer Science Professor Rina Dechter of UC Irvine’s Donald Bren School of Information and Computer Sciences (ICS) is one of five co-principal investigators for a $5 million, multi-institutional National Science Foundation grant titled “Causal Foundations of Decision Making and Learning.”
The grant, which aims to revolutionize AI decision-making by advancing the science of causal inference, is led by Elias Bareinboim of Columbia University. In addition to co-PI Professor Dechter and ICS investigators Roy Fox and Alexander Ihler, the project includes co-PIs and investigators from four other institutions.
“When you’re talking about AI, decision-making is everywhere,” says Dechter. “This will be theoretical, foundational work, but it is applicable to everything, because causality is how people understand the world.” Two real-world use cases motivating the work are robotics and public health, and the goal of the five-year project is to unite the fields of causal inference of AI planning and reinforcement learning, creating a new framework for causal-empowered decision-making in AI.
Building Trust in AI
AI systems that can justify and explain their decisions will increase AI safety and trustworthiness. So, Dechter plans to leverage her expertise in algorithms for probabilistic graphical models and constraint networks, while others bring a wealth of knowledge in the areas of causality and reinforcement learning.
“Right now, learning algorithms are sort of a black box; you cannot understand why they are making this or that suggestion,” says Dechter. The team plans to augment learning algorithms with causality using a model-based paradigm. “When you talk about causality, it means you have some kind of causal model of the world.” Such models can help clarify how an AI system arrives at its decisions. “To develop trust, you have to have an explanation, which is inherent when you have a causal model,” says Dechter. “It can explain the ‘why.’”
It also helps people better understand what level of confidence to place in AI-based decisions. “If you understand how it’s working,” she says, “you can also understand when you need more data.”
A Framework for Causality-Empowered AI
The project will involve outreach and knowledge-transfer activities — such as workshops and mentoring programs — and the bulk of the research will involve three main thrusts.
- Study essential aspects of causal decision-making to ensure autonomous agents are robust, sample-efficient and precise.
- Study aspects of causal decision-making that are important when humans are in the loop, including constructing explanations, deciding when to involve humans, and making fair decisions that align with society’s values and expectations.
- Enhance the scalability of the resulting tools and their ability to reason efficiently.
By developing new principles, theory and algorithms from this research, and evaluating their application in the domains of robotics and public health, a new framework for causal-empowered AI will emerge.
— Shani Murray